Basic pipeline for exploratory analysis#
Load modules#
[1]:
from robustica import RobustICA, corrmats
import pandas as pd
import numpy as np
from sklearn.utils import parallel_backend
import matplotlib.pyplot as plt
import seaborn as sns
Load data from Sastry (2019)#
[2]:
url = "https://static-content.springer.com/esm/art%3A10.1038%2Fs41467-019-13483-w/MediaObjects/41467_2019_13483_MOESM4_ESM.xlsx"
data = pd.ExcelFile(url)
data.sheet_names
[2]:
['README',
'Metadata',
'Expression Data',
'S matrix',
'A matrix',
'Media Recipes',
'Gene Information']
[3]:
data.parse("README")
[3]:
Sheet Name | Description | |
---|---|---|
0 | Experimental Conditions | Experimental conditions for each sample in PRE... |
1 | Expression Data | Expression levels of genes (log2 Transcripts p... |
2 | S matrix | I-modulon gene coefficients. Each column is an... |
3 | A matrix | Condition-specific i-modulon activities. Each ... |
4 | Media Description | Recipes for base media and trace element mixtures |
5 | Gene Information | Mapping of b-numbers to gene names/annotations... |
6 | NaN | NaN |
7 | Metadata Columns | Description |
8 | Sample ID | Unique sample identifier for experiment (e.g. ... |
9 | Study | Short 1-2 word description of study where samp... |
10 | Project ID | Identifier for study |
11 | Condition ID | Identifier for unique experimental conditions |
12 | Replicate # | Suffix for sample ID to distinguish between re... |
13 | Strain Description | Describes gene knock-out, mutation knock-in, o... |
14 | Strain | MG1655 or BW25113 |
15 | Base Media | Media recipe as described in Media Description... |
16 | Carbon Source (g/L) | Carbon source in g/L |
17 | Nitrogen Source (g/L) | Nitrogen source in g/L |
18 | Electron Acceptor | Aerobic (O2), anaerobic (None), or nitrate (KN... |
19 | Trace Element Mixture | Trace element recipe as described in Media Des... |
20 | Supplement | Additional media supplements in each experimen... |
21 | Temperature (C) | Temperature of growth condition in Celsius |
22 | pH | Neutral (7) or acidic (5.5) |
23 | Antibiotic | Treatment with low concentration Kanamycin for... |
24 | Culture Type | Batch or Chemostat (always exponential growth ... |
25 | Growth Rate (1/hr) | Measured growth rate of strain in media |
26 | Evolved Sample | Whether the strain was from an adaptive labora... |
27 | Isolate Type | Clonal or Population (only applicable to ALE s... |
28 | Sequencing Machine | Type of machine used for sequencing |
29 | Additional Details | Additional information about experiment |
30 | Biological Replicates | Number of biological replicates within study |
31 | Alignment | Percent of reads aligned by bowtie to referenc... |
32 | DOI | Accession number for study |
33 | GEO | GEO Accession ID |
Preprocess data#
Following the article’s methods, we compute the log2FCs with respect to controls
[4]:
# get gene expression
X = data.parse("Expression Data").set_index("log-TPM")
# get controls
controls = [c for c in X.columns if "control" in c]
print(controls)
# compute log2FCs with respect to controls
X = X - X[controls].mean(1).values.reshape(-1, 1)
X
['control__wt_glc__1', 'control__wt_glc__2']
[4]:
control__wt_glc__1 | control__wt_glc__2 | fur__wt_dpd__1 | fur__wt_dpd__2 | fur__wt_fe__1 | fur__wt_fe__2 | fur__delfur_dpd__1 | fur__delfur_dpd__2 | fur__delfur_fe2__1 | fur__delfur_fe2__2 | ... | efeU__menFentC_ale29__1 | efeU__menFentC_ale29__2 | efeU__menFentC_ale30__1 | efeU__menFentC_ale30__2 | efeU__menFentCubiC_ale36__1 | efeU__menFentCubiC_ale36__2 | efeU__menFentCubiC_ale37__1 | efeU__menFentCubiC_ale37__2 | efeU__menFentCubiC_ale38__1 | efeU__menFentCubiC_ale38__2 | |
---|---|---|---|---|---|---|---|---|---|---|---|---|---|---|---|---|---|---|---|---|---|
log-TPM | |||||||||||||||||||||
b0002 | -0.061772 | 0.061772 | 0.636527 | 0.819793 | -0.003615 | -0.289353 | -1.092023 | -0.777289 | 0.161343 | 0.145641 | ... | -0.797097 | -0.791859 | 0.080114 | 0.102154 | 0.608180 | 0.657673 | 0.813105 | 0.854813 | 0.427986 | 0.484338 |
b0003 | -0.053742 | 0.053742 | 0.954439 | 1.334385 | 0.307588 | 0.128414 | -0.872563 | -0.277893 | 0.428542 | 0.391761 | ... | -0.309105 | -0.352535 | -0.155074 | -0.077145 | 0.447030 | 0.439881 | 0.554528 | 0.569030 | 0.154905 | 0.294799 |
b0004 | -0.065095 | 0.065095 | -0.202697 | 0.119195 | -0.264995 | -0.546017 | -1.918349 | -1.577736 | -0.474815 | -0.495312 | ... | -0.184898 | -0.225615 | 0.019575 | 0.063986 | 0.483343 | 0.452754 | 0.524828 | 0.581878 | 0.293239 | 0.341040 |
b0005 | 0.028802 | -0.028802 | -0.865171 | -0.951179 | 0.428769 | 0.123564 | -1.660351 | -1.531147 | 0.240353 | -0.151132 | ... | -0.308221 | -0.581714 | 0.018820 | 0.004040 | -1.228763 | -1.451750 | -0.839203 | -0.529349 | -0.413336 | -0.478682 |
b0006 | 0.009087 | -0.009087 | -0.131039 | -0.124079 | -0.144870 | -0.090152 | -0.219917 | -0.046648 | -0.044537 | -0.089204 | ... | 1.464603 | 1.415706 | 1.230831 | 1.165153 | 0.447447 | 0.458852 | 0.421417 | 0.408077 | 1.151066 | 1.198529 |
... | ... | ... | ... | ... | ... | ... | ... | ... | ... | ... | ... | ... | ... | ... | ... | ... | ... | ... | ... | ... | ... |
b4688 | -0.261325 | 0.261325 | -1.425581 | -2.734490 | 0.181893 | 0.514395 | -1.943947 | -1.992701 | 0.066037 | -0.695325 | ... | -0.885297 | -0.462485 | -2.734490 | -1.451148 | -1.379069 | -1.567420 | -0.999610 | -1.726577 | -2.734490 | -1.189069 |
b4693 | -0.278909 | 0.278909 | 1.361362 | 1.020310 | 0.608108 | 0.988541 | 2.558416 | 2.142724 | 3.120867 | 3.104887 | ... | -0.374963 | 0.856574 | -1.147824 | -0.814089 | 2.054471 | 1.853620 | 1.957717 | 1.943582 | 2.233115 | 2.023755 |
b4696_1 | 0.050526 | -0.050526 | 1.166436 | 1.043373 | -0.531441 | -0.581626 | 0.914055 | 0.731165 | -0.127269 | -0.164046 | ... | 0.261604 | 0.278426 | 0.201089 | -0.017780 | 0.138178 | 0.122287 | 0.504402 | 0.425213 | 0.629383 | 0.805945 |
b4696_2 | -0.031653 | 0.031653 | 0.785573 | 0.881353 | -0.477271 | -0.916095 | 0.837603 | 0.801393 | -0.071710 | -0.000540 | ... | -0.499371 | 0.398783 | 0.096609 | -0.103446 | -0.519098 | 0.615363 | 0.343959 | 0.580288 | 0.366905 | 0.702608 |
b4705 | 0.724324 | -0.724324 | -4.350151 | -4.317498 | -0.747489 | -1.257045 | -3.308337 | -4.421970 | -2.679693 | -1.872713 | ... | -1.968530 | -1.365300 | -5.468290 | -2.997169 | -3.673367 | -3.161608 | -3.959910 | -4.088644 | -5.468290 | -5.468290 |
3923 rows × 278 columns
Run robustica
#
Using our version of the Icasso algorithm#
By default, robustica
performs robust ICA by 1. running FastICA multiple times (robust_runs
=100) 2. inferring and correcting the signs of components across runs (robust_infer_signs=True
) 3. compressing the feature space (rows) (robust_dimreduce=True
) 4. using sign-corrected and compressed components to obtain clusters of independent components (robust_method="AgglomerativeClustering"
and robust_kws={"linkage": "average"}
) 5. uses the clustering labels to compute robust independent components
[5]:
%%time
rica = RobustICA(n_components=100, robust_runs=8)
with parallel_backend("loky", n_jobs=4):
S, A = rica.fit_transform(X.values)
print(S.shape, A.shape)
0%| | 0/8 [00:00<?, ?it/s]
Running FastICA multiple times...
100%|██████████| 8/8 [00:03<00:00, 2.24it/s]
Inferring sign of components...
Reducing dimensions...
Clustering...
Computing centroids...
(3923, 100) (278, 100)
CPU times: user 3.71 s, sys: 312 ms, total: 4.02 s
Wall time: 7.6 s
Using the classical Icasso algorithm#
[6]:
%%time
rica = RobustICA(
n_components=100,
robust_runs=8,
robust_infer_signs=False,
robust_dimreduce=False,
robust_kws={"affinity": "precomputed", "linkage": "average"},
)
with parallel_backend("loky", n_jobs=4):
S_classic, A_classic = rica.fit_transform(X.values)
print(S_classic.shape, A_classic.shape)
0%| | 0/8 [00:00<?, ?it/s]
Running FastICA multiple times...
100%|██████████| 8/8 [00:02<00:00, 3.09it/s]
Precomputing distance matrix...
Clustering...
Computing centroids...
(3923, 100) (278, 100)
CPU times: user 2.38 s, sys: 132 ms, total: 2.51 s
Wall time: 7.51 s
Correspondence between the two approaches#
[7]:
plt.figure(figsize=(11, 10))
sns.heatmap(
np.abs(corrmats(S.T, S_classic.T)),
cmap="coolwarm",
center=0,
cbar_kws={"label": r"$abs(Pearson Correlation)$"},
)
plt.xlabel("Classic ICs")
plt.ylabel("Default ICs")
plt.show()
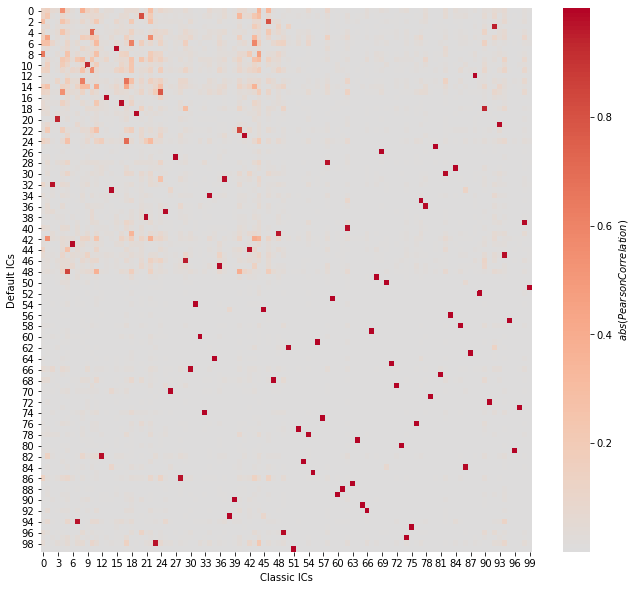